Identifying User by Collaborative Statistics: A Review
Rasika Retar, Pratiksha Dhoble, Abhishek Chauhan, Swati Gourkhede, Bhavna Sonkusre, Prof. Pradeep O. Balbudhe
Volume 5: Issue 1, March 2018, pp 1-4
Author's Information
Prof. Pradeep O. Balbudhe1
Corresponding Author
1Assistant Professor, Department Of Computer Engineering, Suryodaya College Of Engineering & Technology, Nagpur, Maharashtra, India
Pb6143@gmail.com
Rasika Retar, Pratiksha Dhoble, Abhishek Chauhan, Swati Gourkhede, Bhavna Sonkusre
2Students, Department Of Computer Engineering, Suryodaya College Of Engineering & Technology, Nagpur, Maharashtra, India
Abstract:-
Today’s we have many as n numbers of online services. Those services use by most of users. Every user has their own unique behaviour or pattern of uses. The users behaviour or we can say usage pattern which can we are going to give a feat by tracking there usage of online services for identifying the user statistically. We are using a powerful algorithm which can help us to identify the user by time, location, as worldwide and which give a output with the help of histogram as a graph.As user identities of both the database which a database of source one and source two as mention above, then this is a insignificant. A common requirement in our database is to analyzing for identifying user by a feat statistics of their data which we are going to actually work on.
Index Terms:-
ICS system, Web User, Data Flow Diagram, Algorithm.REFERENCES
[1] Farid M. Naini, Jayakrishnan Unnikrishnan, Patrick Thiran and Martin Vetterli, “Where you are is who you are: user identification by matching statistic”,(volume: 11,issue: 2),05 November 2016.[2] Joonseok Lee, Mingxuan Sun, Guy Lebanon, “A Comparative Study of Collaborative Filtering-Algorithms.” 2012.
[3] Reena Pagare, Shalmali A. Patil, “Study of Collaborative Filtering Recommendation Algorithm-Scalability Issue” international journal of computer Application(0975-8887)Volume 67-No. 25, April 2013.
[4] Peng Peng, Hu Bin, “A Collaborative Filtering Recommendation Based on User Characteristics and Time Weight[J]”,Journal of Wuhan University of Technology, vol. 31, no.3, pp. 24-28, 2009.
[5] Huang Huang, Wei Wei, Ye Ye et al.,”Collaborative Filtering Algorithm Based on User property and item Category[J]”,Computer & Digital Engg., vol. 40, no.10,pp.5-7,2012.
[6] Zha Zha, Li Li, Xu Guiqiong, ”An Optimised Collaborative Filtering Algo Base on Combined Similarity[J]”, Computer Applications and Software, vol. 31, no.12, pp 323-328,2014.
[7] Wu Wu, Wang Haoran,”Collaborative Filtering Algo Using User Background Information[J]”, Computer Application, vol. 28, no. 11, pp .58-60, 2008.
[8] P B K kantor, L Rokach, F Ricci et al., “Recommender Sys handbook[M],” RAMEPublishers, 2011.
[9] L Lu, M Medo, C H Yeung et al., “Recommender sys[J]”,Physics Reports, Vol. 519,no. 1, pp. 1-49,2012.
To view full paper, Download here
To View Full Paper
For authors
Author's guidelines Publication Ethics Publication Policies Artical Processing Charges Call for paper Frequently Asked Questions(FAQS) View all Volumes and IssuesPublishing with
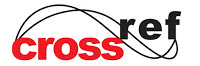
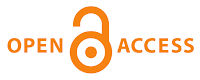
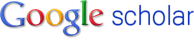
